If you’ve ever asked your phone to play your favourite song or typed a quick question into a search engine, you’ve already met artificial intelligence. AI seems so ordinary now—almost like it’s been quietly living in our pockets for years, waiting for us to wake up to its power. And yet, whenever I talk to friends and family about AI, they often say, “Well, it’s just machines doing their thing, right?” I wish it were that simple.
Have you ever wondered who trains these AI systems or how they learn about the world? That, my friend, is where AI ethics and bias pop into the picture. Because when AI gets stuff wrong—or even when it seems to get stuff right—there’s always a bigger story behind it.
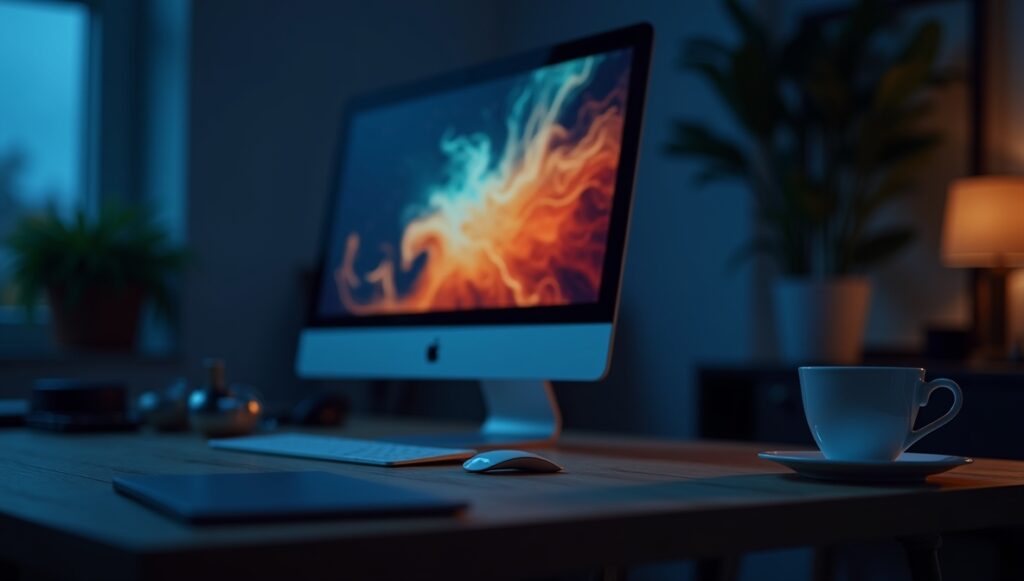
Understanding AI Ethics and Bias
I recall a friend once telling me about applying for a new job. He sent in his CV online, and an automated system scanned it. Moments later, he was met with a polite rejection email—no interview, no phone call, no explanation. We can’t say for certain whether it was AI that ruled him out. But plenty of hiring platforms do use AI to filter candidates, and that’s where ethical considerations come into play.
When AI systems are taught using data that’s skewed—for example, historical hiring data that favoured certain types of candidates—they carry that bias into the future. Imagine you’re teaching a toddler about colours. If you only ever show them red and green, they’ll learn that those two colours exist, but they might not have the faintest clue that purple or turquoise are part of the rainbow. This is exactly how AI systems can miss entire segments of the population if their training data is incomplete or skewed. It’s worrying, isn’t it?
The stakes go beyond hiring decisions. From credit scoring to medical diagnosis, AI’s biases can subtly and repeatedly disadvantage people from particular communities. Sometimes it’s not even intentional—these systems are often black boxes of pattern-recognition, but guess what? They absorb all the cracks and flaws in our society. We need empathy.
The Human Impact of Bias
Let’s explore a hypothetical scenario: Suppose your local hospital starts using an AI-driven tool to prioritise patients for emergency care. It might consider factors like age, past medical records, and frequency of hospital visits. Maybe the data it was trained on came mostly from urban areas. Suddenly, older people in rural spots might be overlooked if they haven’t seen a doctor as frequently—unfair, right?
It feels unfair because it is. Bias in AI doesn’t always announce itself. Instead, it sneaks into corners where few people bother to check. This silent infiltration is what keeps me awake some nights… because the repercussions can be massive.
Thankfully, we’re seeing efforts to curb these issues. The European Union has been drafting regulations to hold AI developers accountable for the data they use. Meanwhile, local advocacy groups—and even small AI startups—are drawing attention to the silent weight of AI bias. If you’ve been following the news, you’ve probably seen glimpses of these conversations, though they might not always make the front page.
The Latest Research: Niche but Vital
Industry insiders often talk about mainstream reports from big names, but sometimes the smaller, niche research unveils the real story. Earlier this year, Hugging Face released an LLM adoption survey that dug into how organisations of different sizes were training their language models. The results? It wasn’t just that large companies had bigger datasets; it was that smaller companies were relying heavily on community-generated data. While this approach fosters collaboration, it can also introduce a patchwork of biases from various unfiltered data sources.
This survey might not have made mainstream headlines, yet it underscores the very heart of the AI ethics debate—who controls the data and how well is it cleaned up? Hugging Face pointed out that many respondents felt uncertain about the reliability of these open datasets, highlighting the urgent need to establish stronger ethical frameworks.
Why Transparency Matters
So, where does that leave us? One of the main reasons AI ethics is so complex is because machine learning is, by nature, a bit of a mystery. Unless companies are totally transparent about how they gather and train their models, everyday people won’t know how decisions are being made—nor will we know if those decisions are fair.
Think of AI models like a secret recipe for baking bread. You get a crusty loaf at the end, but without seeing the chef measure and mix the ingredients, you don’t really know why one loaf might taste slightly off. And if the chef accidentally adds salt instead of sugar, the entire loaf changes its flavour. That’s precisely why we need transparency, audits, and possibly even third-party checks to prevent that accidental salt from spoiling the recipe.
Being Proactive and Practical
Now, I’m not suggesting we scrap AI altogether. In fact, I’m a huge advocate for harnessing AI’s potential for good. Tools that help doctors spot diseases earlier or assist farmers in optimising crop yields can do wonders for humanity—so long as they’re developed with a sense of responsibility.
Individuals can do their part as well. If you’re working with AI in any capacity, speak up about data quality and diversity. It might feel a bit awkward, but trust me, it’s crucial. Managers and CEOs rarely come forward to say “We’re cutting corners!” but you can gently nudge them to consider the ethical ramifications of their choices.
Looking Ahead
The conversation around AI ethics and bias isn’t going anywhere. If anything, it’s gaining momentum as governments, researchers, and tech companies scramble to address the moral questions that arise from every new AI development. Just last week, I read about newly proposed guidelines in the UK seeking to hold automated decision-makers accountable for misinformation. They’re not perfect. But it’s a start.
All of this signals a shift from a “move fast and break things” culture—famous in Silicon Valley—to one that prioritises trust, fairness, and empathy. It’s about time, really.
Conclusion
AI ethics isn’t just about advanced math or coding. It’s about people, their fears, dreams, and daily struggles. Every data point that goes into an algorithm represents someone’s story or experience, so we owe it to ourselves—and to future generations—to get this right. By staying informed, questioning the status quo, and pushing for transparency, we can ensure AI remains a tool that empowers us rather than one that reinforces old biases.
I’d love to hear your thoughts—because, in the end, AI is about all of us. After all, the more we talk about these issues, the better our chances of creating a future we can all be proud of.